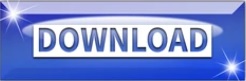
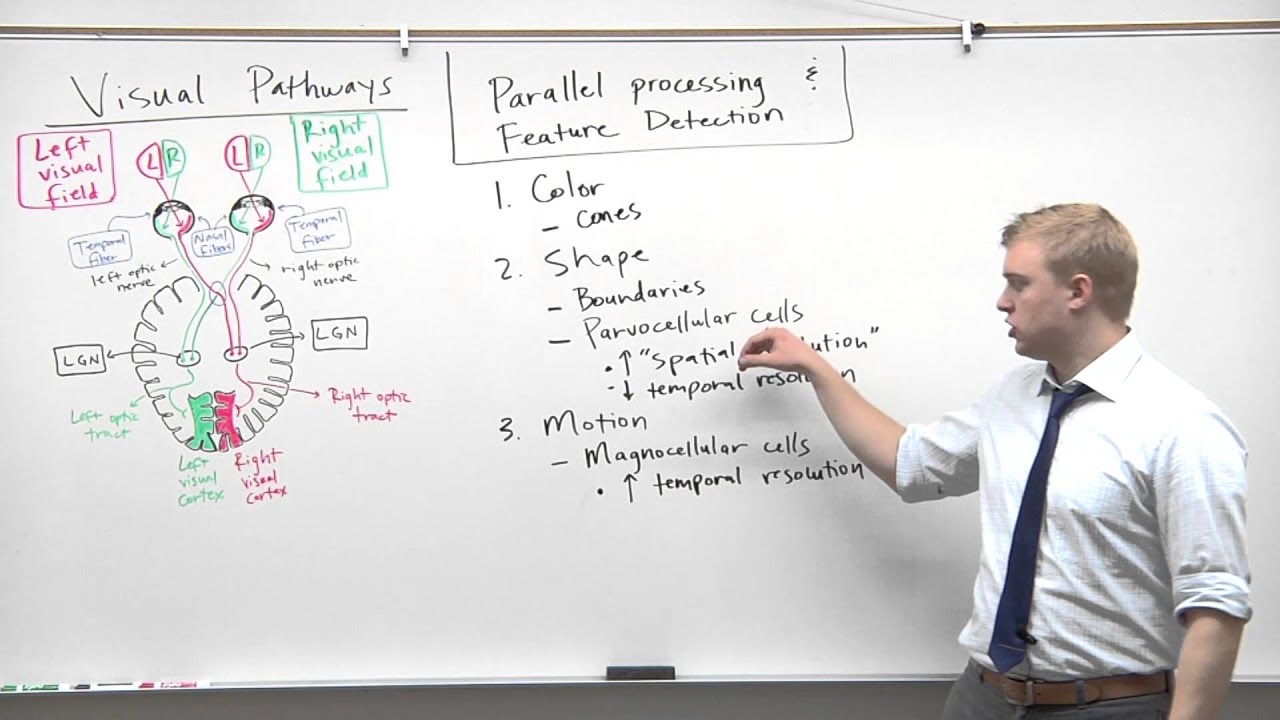
At any one time only a single thread can acquire a lock for a Python object or C API. This means that there is a globally enforced lock when trying to safely access Python objects from within threads. The GIL is necessary because the Python interpreter is not thread safe. Unfortunately the internals of the main Python interpreter, CPython, negate the possibility of true multi-threading due to a process known as the Global Interpreter Lock (GIL).
#Eventscripts parallel processing code#
The expectation is that on a multi-core machine a multithreaded code should make use of these extra cores and thus increase overall performance. One of the most frequently asked questions from beginning Python programmers when they explore multithreaded code for optimisation of CPU-bound code is "Why does my program run slower when I use multiple threads?". In particular we are going to consider the Threading library and the Multiprocessing library. Monte Carlo simulations used for options pricing and backtesting simulations of various parameters for algorithmic trading fall into this category. These models work particularly well for simulations that do not need to share state.
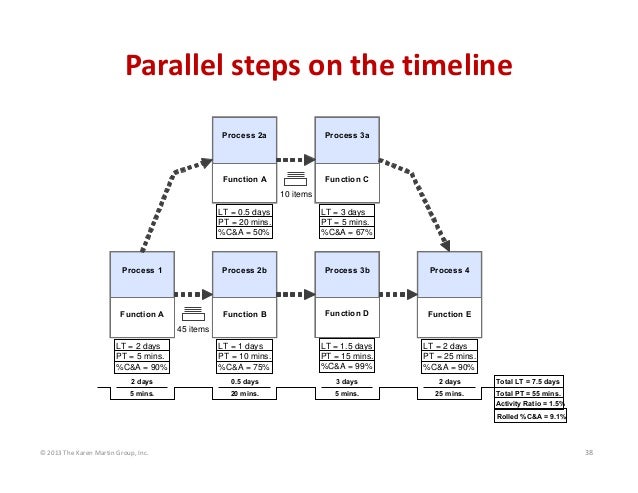
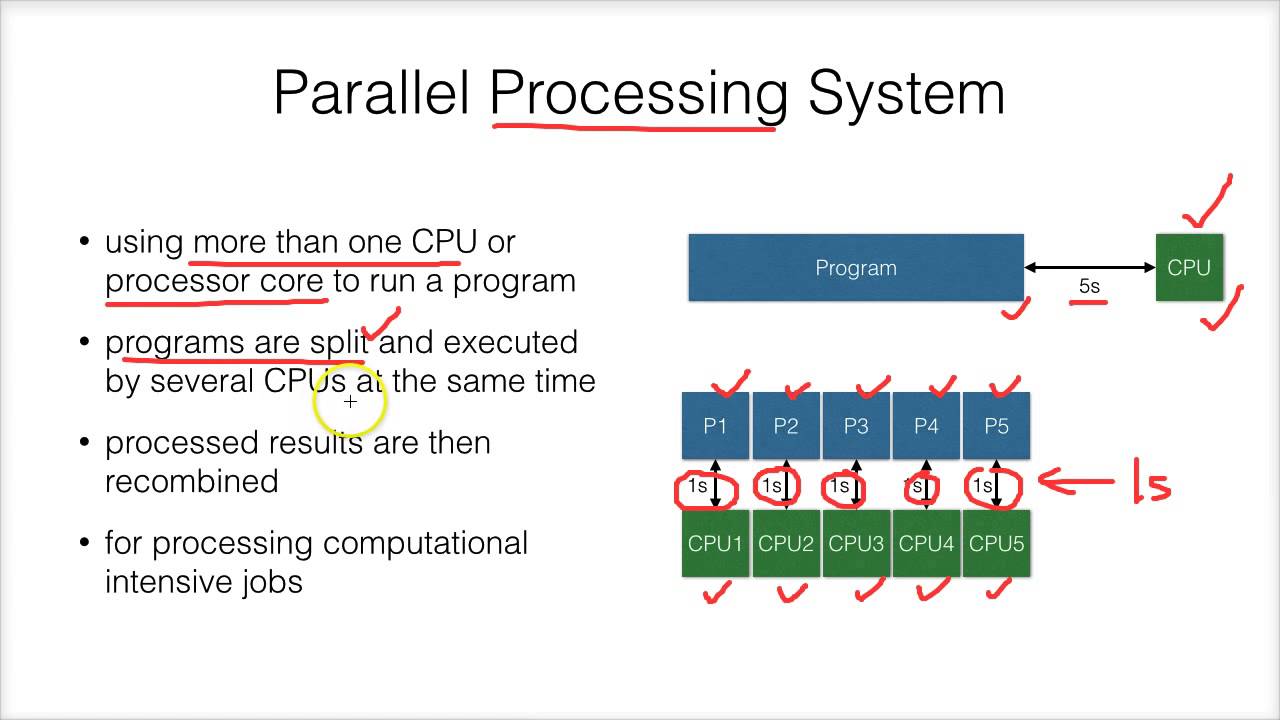
In this article we are going to look at the different models of parallelism that can be introduced into our Python programs. Are there any other means available to us to speed up our code? The answer is yes - but with caveats! While NumPy, SciPy and pandas are extremely useful in this regard when considering vectorised code, we aren't able to use these tools effectively when building event-driven systems.
#Eventscripts parallel processing how to#
Every iteration of the loop can overlap with any other iteration, although within each loop iteration there is little opportunity for overlap.One aspect of coding in Python that we have yet to discuss in any great detail is how to optimise the execution performance of our simulations. While pipelining is a form of ILP, we must exploit it to achieve parallel execution of the instructions in the instruction stream. Instruction-level parallelism means the simultaneous execution of multiple instructions from a program.
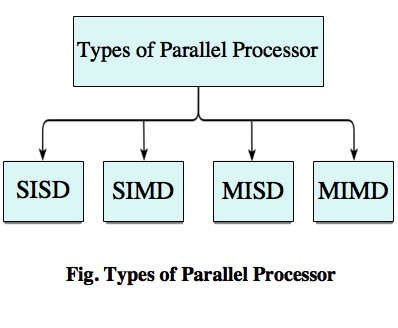
A processor with 16- bit would be able to complete the operation with single instruction. First the 8 lower-order bits from each integer were must added by processor, then add the 8 higher-order bits, and then two instructions to complete a single operation. In this type of parallelism, with increasing the word size reduces the number of instructions the processor must execute in order to perform an operation on variables whose sizes are greater than the length of the word.Į.g., consider a case where an 8-bit processor must add two 16-bit integers. Bit-level parallelismīit-level parallelism is a form of parallel computing which is based on increasing processor word size. Again The threads are operating in parallel on separate computing cores, but each is performing a unique operation. Task Parallelism means concurrent execution of the different task on multiple computing cores.Ĭonsider again our example above, an example of task parallelism might involve two threads, each performing a unique statistical operation on the array of elements. So the Two threads would be running in parallel on separate computing cores. and while thread B, running on core 1, could sum the elements. For a dual-core system, however, thread A, running on core 0, could sum the elements. For a single-core system, one thread would simply sum the elements. Let’s take an example, summing the contents of an array of size N. Data Parallelism means concurrent execution of the same task on each multiple computing core.
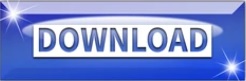